Authors: Caitlin Munro, PharmD (Senior Manager, Clinical Programs), and Zach Brunko, PharmD (PGY-1 Managed Care Resident)
In Part 2 of GLP-1s, What We Know Now, For Now, we address how, and to what extent, payers will materially differentiate between patient segments. We also explain what share of the market is expected to be reimbursed versus cash.
While it is unclear how payers may assign value to different patient segments, data has shown that social determinants of health (SDOH) could be a key differentiator. Other strategies may involve focusing on achieving, sustaining, and tracking toward various treatment goals such as weight loss and improved cholesterol or blood pressure control. Interpreting prior authorization data could also help determine what inferred, potential percentage of the market may be paying out-of-pocket for weight loss agents in the current reimbursement landscape.
How Social Determinants of Health (SDOH) and BMI Are Shaping Patient Segmentation
As mentioned above, potential segmentation of patients could be driven by social determinants of health (SDOH) including housing, disability, geography, and income12,13. For instance, a study found that Medicare/Medicaid covered patients with lower education and income levels were more likely to be considered obese12. In some studies, patients with higher SDOH burden were 70% more likely to be considered obese and overweight12.
Body mass index (BMI) has also been used to segment patients as both a gauge for overall health and as part of criteria for medication coverage. However, physicians and other healthcare providers argue that BMI may not accurately reflect the health of a patient. For instance, BMI is associated with varying degrees of risk for developing diabetes or increased mortality rate across different age, ethnicity and gender groups observed13,14.
Other strategies to differentiate patients into segments include performance-based assessments centered around patients achieving, maintaining, and tracking health goals.
Qualitative findings, as seen in the example below, can be used to segment patients based on, for instance, an assessment on if patients are meeting their goals or not15.

Market Reimbursement vs. Out-of-Pocket Payments: Insights from Prior Authorization Data
The share of the market expected to be reimbursed as part of expanded AOM coverage versus the share to pay out-of-pocket (cash) could be inferred by extrapolating Capital Rx prior authorization approval and denial data accessed via Tableau.
When looking at AOMs across all lines of business, a nearly even 50/50 split of AOM approvals and denials occurred between January 1, 2023 to June 23, 2024. A further breakdown highlights Wegovy and Zepbound16.

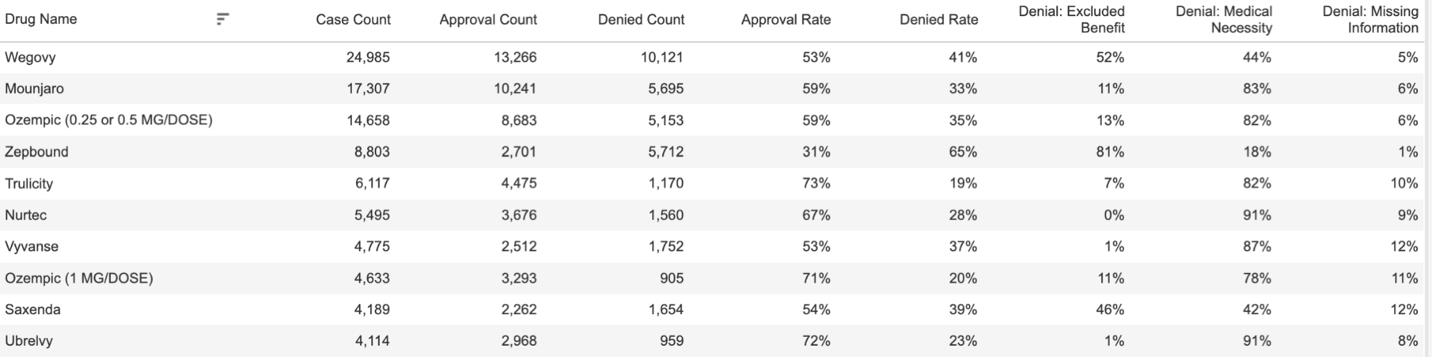
Based on the prior authorization data observed, an assumption can be made that some patients may consider paying out-of-pocket for denied AOM claims.
The data observed may not capture all patients who pay out-of-pocket for their AOM as some may forgo the prior authorization process. However, considering the high-cost patients will incur by paying the out-of-pocket retail price for GLP-1 medications, many may steer away from therapy altogether.
Resources
- Racca J. Can using SDOH data help identify patient populations who could benefit from weight loss drugs? PurpleLabTM Healthcare Analytics. Available from: https://purplelab.com/can-using-sdoh-data-help-identify-patient-populations/. Published May 17, 2024.
- Javed Z, Valero-Elizondo J, Maqsood MH, Mahajan S, Taha MB, Patel KV, Sharma G, Hagan K, Blaha MJ, Blankstein R, Mossialos E, Virani SS, Cainzos-Achirica M, Nasir K. Social determinants of health and obesity: Findings from a national study of US adults. Obesity (Silver Spring). 2022 Feb;30(2):491-502. doi: 10.1002/oby.23336. PMID: 35088551.
- National Academies of Sciences, Engineering, and Medicine; Health and Medicine Division; Food and Nutrition Board; Roundtable on Obesity Solutions; Berhaupt A, editor. Exploring the Science on Measures of Body Composition, Body Fat Distribution, and Obesity: Proceedings of a Workshop Series. Washington (DC): National Academies Press (US); 2024 Mar 20. 6, Communicating How Obesity Is Defined and Diagnosed. Available from: https://www.ncbi.nlm.nih.gov/books/NBK603298/
- Bobinet, K and Greer, S. The Iterative Mindset Method: a neuroscientific theoretical approach for sustainable behavior change and weight-loss in digital medicine. NPJ Digital Medicine 6(1). DOI:10.1038/s41746-023-00910-y. Published Sep, 2023.
- Tableau® Online. Prior Authorization. Updated Jul, 2024. Accessed Jul, 2024.